The world of machine learning is constantly evolving, with technology advancing at an unprecedented pace. One of the most innovative and game-changing developments in this field is the use of synthetic data generation. But what exactly is synthetic data generation, and how does it revolutionize machine learning? In this blog post, we will delve deep into the power of synthetic data generation and explore how it has the potential to transform the way we approach machine learning algorithms.
Imagine a scenario where you have limited access to real-world data for your machine-learning project. Perhaps the data is sensitive, expensive to collect, or simply not available. This is where synthetic data generation comes into play, offering a solution that unlocks the power of limitless data. By using complex algorithms and statistical models, synthetic data generation allows researchers and developers to create artificial datasets that closely mimic real-world data. These artificially generated datasets can then be used to train machine learning models, offering a realistic and diverse range of examples for the algorithms to learn from.

But why is synthetic data generation such a game-changer? Well, imagine training a self-driving car without exposing it to countless hours of real-world driving scenarios. Or training a medical diagnosis system without access to a vast number of patient records. Synthetic data generation fills these gaps, empowering machine learning algorithms to learn from a wide range of scenarios, even ones that are difficult to obtain in the real world. In this blog post, we will explore the techniques and benefits of synthetic data generation, as well as its limitations and challenges.
We will also discuss real-world examples where SDG has already made a significant impact in various industries. So, fasten your seatbelts and get ready to dive into the world of synthetic data generation, where the possibilities for revolutionizing machine learning are truly endless.
The Basics of Synthetic Data Generation
Synthetic data generation is a powerful technique that has the potential to revolutionize machine learning. In this section, we will explore the fundamentals of synthetic data generation and how it works. At its core, synthetic data generation involves creating artificial datasets that closely resemble real-world data. This is achieved through the use of complex algorithms and statistical models. These algorithms analyze existing datasets to identify patterns and relationships between variables. Once these patterns are identified, the algorithms can generate new data points that adhere to these patterns.
There are several techniques used in SDG, including random sampling, interpolation, and generative adversarial networks (GANs). Random sampling involves randomly selecting values from existing datasets to create new data points. Interpolation involves estimating missing values based on known values in the dataset. GANs are a more advanced technique that involves training two neural networks: a generator network that creates synthetic data and a discriminator network that distinguishes between real and synthetic data.
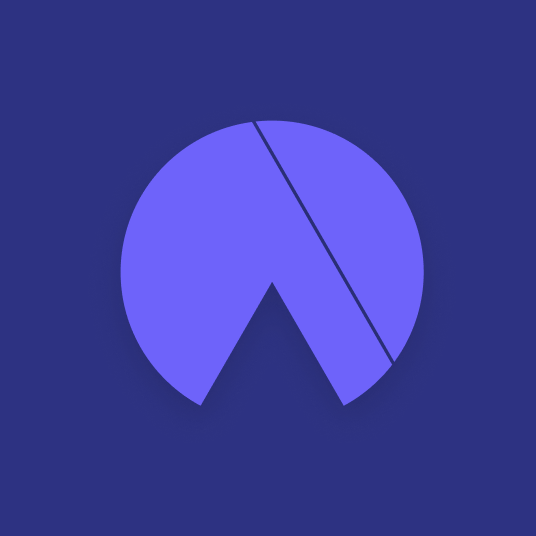
Understanding the Power of Limitless Data
One of the key advantages of synthetic data generation is its ability to provide limitless amounts of training data for machine learning algorithms. In many cases, obtaining real-world data can be challenging due to factors such as privacy concerns or limited availability. Synthetic data generation overcomes these limitations by allowing researchers and developers to generate as much artificial data as they need.By having access to vast amounts of diverse training examples, machine learning algorithms can learn more effectively and make better predictions. This is particularly beneficial in scenarios where collecting large amounts of real-world data is impractical or costly.
Techniques for Synthetic Data Generation
As mentioned earlier, there are several techniques used in synthetic data generation. Let's take a closer look at each technique:
1. Random Sampling:
This technique involves randomly selecting values from existing datasets to create new artificial data points. The randomness ensures that the generated dataset closely resembles the original dataset in terms of statistical properties.
2. Interpolation:
Interpolation is used when there are missing values in the dataset. This technique estimates the missing values based on known values and the relationships between variables. By filling in the gaps, the synthetic dataset becomes more complete and representative of the real-world data.
3. Generative Adversarial Networks (GANs):
GANs are a more advanced technique that involves training two neural networks simultaneously. The generator network creates synthetic data, while the discriminator network tries to distinguish between real and synthetic data. Through an iterative process, both networks improve their performance, resulting in high-quality synthetic datasets.
Benefits of Using Artificial Datasets
The use of artificial datasets generated through synthetic data generation offers several benefits:
1. Privacy Protection:
In many cases, real-world datasets contain sensitive information that needs to be protected. Synthetic data generation allows researchers to create artificial datasets that do not compromise privacy while still providing valuable training examples.
2. Cost-Effectiveness:
Collecting large amounts of real-world data can be expensive and time-consuming. Synthetic data generation provides a cost-effective alternative by allowing researchers to generate as much artificial data as they need without incurring additional costs.
3. Data Diversity:
Real-world datasets may not always capture the full range of possible scenarios or variations in the data distribution. Synthetic data generation enables researchers to create diverse datasets that cover a wide range of scenarios, improving the robustness and generalization capabilities of machine learning models.

Real-world Applications of Synthetic Data Generation
Synthetic data generation has already found applications in various industries and domains. Let's explore some real-world examples:
1. Healthcare:
In healthcare, access to patient records for research purposes can be limited due to privacy concerns. Synthetic data generation allows researchers to create artificial patient records that closely resemble real ones, enabling them to develop and test new medical diagnosis systems without compromising patient privacy.
2. Autonomous Vehicles:
Training self-driving cars requires exposure to countless hours of real-world driving scenarios. Synthetic data generation can provide a solution by generating artificial driving datasets that simulate various road conditions, traffic scenarios, and weather conditions.
3. Fraud Detection:
Synthetic data generation can be used to create artificial datasets that mimic fraudulent transactions. These datasets can then be used to train fraud detection algorithms, enabling financial institutions to better identify and prevent fraudulent activities.
Overcoming Limitations and Challenges
While synthetic data generation offers many benefits, it also comes with its own set of limitations and challenges. One major challenge is ensuring that the generated synthetic data accurately represents the real-world data distribution. If the synthetic data does not accurately capture the underlying patterns and relationships in the real-world data, it may lead to biased or inaccurate machine-learning models. Another challenge is evaluating the quality of synthetic datasets. It is important to have metrics and evaluation techniques in place to assess how well the synthetic data represents the real-world data.
Ethical Considerations in Synthetic Data Generation
The use of synthetic data raises ethical considerations, particularly when it comes to privacy and consent. It is crucial to ensure that any personal or sensitive information in the original dataset is properly anonymized or removed before generating synthetic data. Additionally, transparency and accountability are important when using synthetic data for decision-making processes. Users should be aware that they are interacting with artificial datasets rather than real ones.
The Future of Machine Learning and Synthetic Data Generation
As machine learning continues to advance, so does the potential for synthetic data generation. The future holds exciting possibilities for this technology, including improved algorithms for generating more realistic and diverse datasets. Furthermore, advancements in deep learning techniques such as GANs will likely lead to even more sophisticated methods of generating high-quality synthetic data.
Conclusion
In conclusion, synthetic data generation has emerged as a powerful tool in revolutionizing machine learning. By providing access to limitless amounts of diverse training examples, it enables machine learning algorithms to learn more effectively and make better predictions. We explored the basics of synthetic data generation, including its techniques, benefits, and real-world applications.
As we look to the future, it is clear that synthetic data generation will continue to play a crucial role in advancing machine learning and unlocking its full potential. With ongoing research and development, we can expect even more exciting applications and advancements in this field. So, embrace the power of synthetic data generation and get ready to witness the transformative impact it can have on machine learning.
Affiliate Disclosure
Prime Se7en may contain affiliate links. This means that if you click on one of these links and make a purchase or sign up for a service, we may receive a commission or referral fee at no additional cost to you. Read more in our Guidelines.
You do not have permission to write comment on this post.
Log in Register